Lecturer Dr. Jonathan Resop was awarded an Instrumentation Discovery Travel Grant (IDTG) from the Consortium of Universities for the Advancement of Hydrologic Science (CUAHSI):
https://www.cuahsi.org/education/instrumentation-discovery-travel-grants/
The grant "enables scientists to learn the details of hydrologic instrument installation, operation, maintenance, and data processing by visiting experts" and will be used by Dr. Resop to visit the StREAM Lab at Virginia Tech, run by Dr. Cully Hession, to learn more about their drone-base lidar system and gain experience using it in the field for data collection:
https://vtstreamlab.weebly.com/
The StREAM Lab's equipment includes a Vapor35 drone and a YellowScan lidar:
https://vtstreamlab.weebly.com/equipment.html
The site visit will be used to enhance Dr. Resop's courses in the MPSGIS Program (GEOG660: Advanced Remote Sensing using Lidar) as well as further research collaboration between Dr. Resop and Dr. Hession in using drone-based lidar for estimating flow roughness metrics. Initial findings from this research will be presented by Dr. Resop at the upcoming 8th International Symposium on Environmental Hydraulics (ISEH) at the University of Notre Dame, June 4-7, 2018:
Title:
"Quantifying Stream and Floodplain Roughness using a Drone-based Lidar and Continuous Flow Monitoring:
Abstract:
Physical measures of riverscapes, such as channel geometry, floodplain connectivity, and spatial complexity are important for a range of ecological and hydromorphological processes. Hydraulic roughness due to channel physical characteristics and vegetation can be a difficult metric to quantify. Roughness directly effects hydraulic properties that influence aquatic habitat, flooding, sediment transport, water quality, and channel morphology. Roughness itself can be classified with respect to its components at different stream stages, such as surface bed material and vegetation. Traditional measures of stream roughness include manual surveys of grain size and vegetative cover, comparisons to images of streams with known roughness, and back calculations from onsite flow measurements. Our objective was to use high-resolution, drone-based lidar to estimate roughness at different stages in a more quantitative and direct manner. Drone-based lidar, or drone laser scanning, combines the surveying capabilities of lidar with the mobility of unmanned aerial vehicles, producing high-density point clouds with the resolution of terrestrial laser scanning and the coverage of aerial laser scanning. We created a data-processing pipeline for processing drone-based lidar to calculate measures of surface roughness (based on the variability of the terrain) and vegetative roughness (based on the variability of the vegetation). We quantified these roughness measures for different flow stages and across seasons (reflecting changes in vegetation). In addition, the roughness measures estimated with drone-based lidar data were validated using continuously-measured flow and stage data at the Virginia Tech StREAM Lab along Stroubles Creek in Blacksburg, VA.
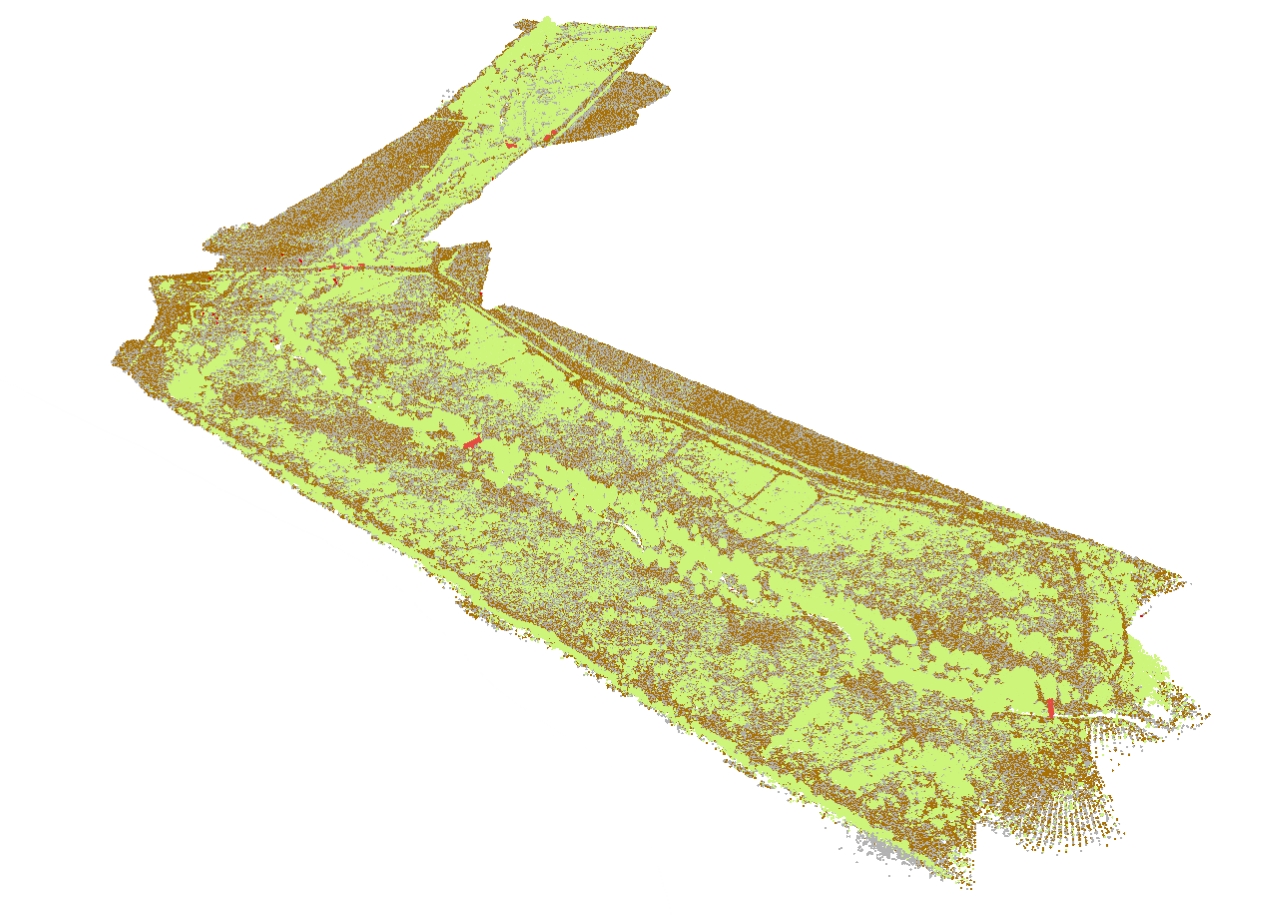