GEOG researchers (UMD PI: Dr. S. Skakun, Co-I: Dr. L. De Floriani) in collaboration with Kiware, Inc., were selected for funding within the NASA 2018 Small Business Innovation Research (SBIR) Program Phase I. The NASA SBIR program funds the research, development, and demonstration of innovative technologies that fulfill NASA needs and have significant potential for successful commercialization. The joint UMD/Kitware project is titled "Open-Source Deep Learning Classification and Visualization of Multi-Temporal Multi-Source Satellite Data" and was submitted within the subtopic "Machine Learning and Deep Learning for Science and Engineering".
Technical Abstract
Availability of high-resolution big data, specifically from Sentinel-1A/B, Sentinel-2A/B, and Landsat-8, presents unprecedented opportunities for a wide range of preoperational and operational applications in the environmental and agricultural domains which will take advantage of these high spatial and temporal resolution datasets as well as advances in multi-source data fusion techniques. One such application is land cover classification and the construction of crop type maps. These maps serve as critical inputs in performing environmental and agriculture monitoring tasks, for instance in case of NASA’s Land Cover Land Use Change (LCLUC) program.
Current popular approaches use deep learning models for remote sensing and utilize a single date image for classification purposes. However, to identify specific land cover classes such as crop types reliably, multi-temporal images are usually required. Use of a multi-temporal-images-based approach presents challenges that include the need to leverage heterogeneous data sources with multiple scales (spatial and temporal), multiple spectral bands, continuous and point-based coverage and incorporating domain knowledge to DL models
To address these challenges and to create an end-to-end deep learning system for crop type classification and characterization for stakeholders to use, we propose to develop DeepRES - an Open Source Deep Learning Platform for REmote Sensing Data. The DeepRES learning network will use multi-temporal multi-source satellite data (EO and SAR), the state-of-the-art in deep learning (Convolutional and Recurrent Neural Networks), scientific data management and visualization products, and Kitware- and community-developed open-source tools such as Girder, TensorFlow, and GeoJS.
DeepRES will benefit stakeholders that are not domain scientists by giving them access to cutting-edge algorithms and benefit data scientists by letting them develop and fine-tune their models using cloud-based infrastructure.
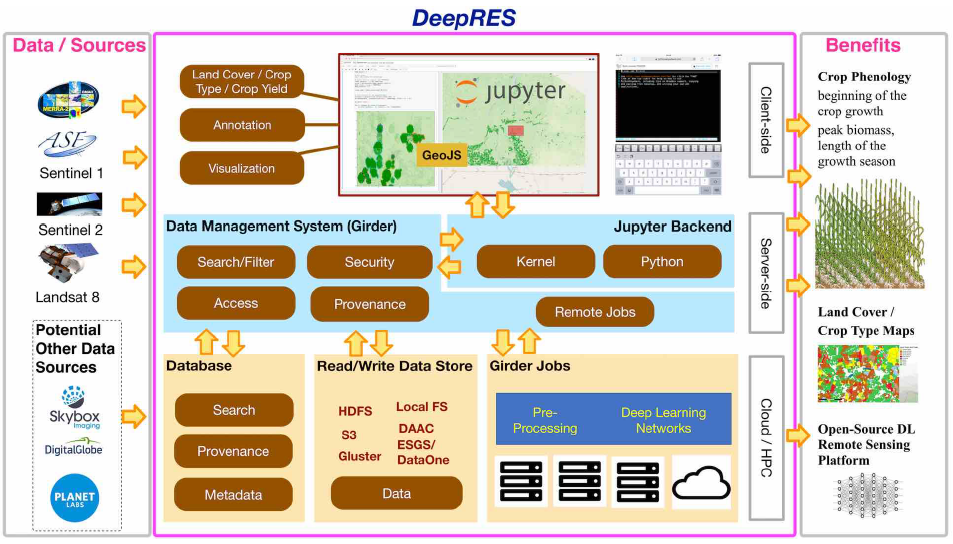