Ritvik Sahajpal, César Izaurralde and George Hurtt along with colleagues from JGCRI and MSU, published an article entitled 'Identifying representative crop rotation patterns and grassland loss in the US Western Corn Belt' in the forthcoming October, 2014 issue of Journal of Computers and Electronics in Agriculture.
The article is available here. The abstract is as follows:
Crop rotations (the practice of growing crops on the same land in sequential seasons) reside at the core of
agronomic management as they can influence key ecosystem services such as crop yields, carbon and
nutrient cycling, soil erosion, water quality, pest and disease control. Despite the availability of the
Cropland Data Layer (CDL) which provides remotely sensed data on crop type in the US on an annual
basis, crop rotation patterns remain poorly mapped due to the lack of tools that allow for consistent
and efficient analysis of multi-year CDLs. This study presents the Representative Crop Rotations Using
Edit Distance (RECRUIT) algorithm, implemented as a Python software package, to select representative
crop rotations by combining and analyzing multi-year CDLs. Using CDLs from 2010 to 2012 for 5 states in
the US Midwest, we demonstrate the performance and parameter sensitivity of RECRUIT in selecting
representative crop rotations that preserve crop area and capture land-use changes. Selecting only 82
representative crop rotations accounted for over 90% of the spatio-temporal variability of the more than
13,000 rotations obtained from combining the multi-year CDLs. Furthermore, the accuracy of the crop
rotation product compared favorably with total state-wide planted crop area available from agricultural
census data. The RECRUIT derived crop rotation product was used to detect land-use conversion from
grassland to crop cultivation in a wetland dominated part of the US Midwest. Monoculture corn and
monoculture soybean cropping were found to comprise the dominant land-use on the newly cultivated
lands.
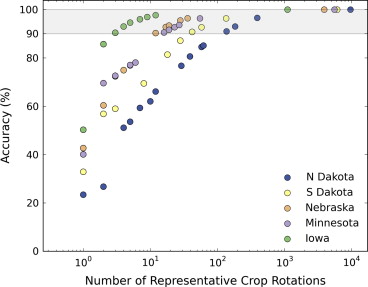